Generative AI: Unleashing Creative Potential
Generative AI, known for its ability to autonomously create content, requires a nuanced evaluation approach. Assessing the creativity and coherence of generated content, while also considering ethical implications, is vital. Evaluation metrics include the diversity of generated outputs, the model’s ability to understand context, and its adherence to predefined guidelines or constraints.
Computer Vision: Interpreting Visual Information
In the realm of Computer Vision, AI systems analyze and interpret visual data, ranging from image recognition to object detection. Evaluating these solutions involves testing their accuracy in identifying objects, people, or patterns within images or videos. Ensuring robustness against variations in lighting conditions, image quality, and diverse perspectives is critical to determining the reliability of Computer Vision models.
Natural Language Processing (NLP): Understanding Context and Semantics
NLP plays a pivotal role in AI solutions that involve language understanding and generation. Evaluating NLP models includes assessing their comprehension of context, semantics, and their ability to generate coherent and contextually relevant responses. Additionally, evaluating language models for bias and sensitivity to potentially harmful inputs is crucial to ensuring ethical and fair use.
Recommendation Systems: Personalizing User Experiences
AI-driven recommendation systems analyze user behavior to suggest personalized content. Evaluation in this domain involves measuring the system’s accuracy in predicting user preferences, the relevance of recommendations, and adaptability to evolving user tastes. Balancing the need for personalization with user privacy considerations is integral to a comprehensive evaluation.
Contact
93, MDC-Sector 5, Panchkula 134114, Haryana, India
info@bnsenterprise.com
manager@bnsenterprise.com
Stay Connected with Us
+91 7087307404
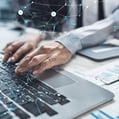